Unfenced pitched questions to three experts, to dig deeper into testing tips and theories.
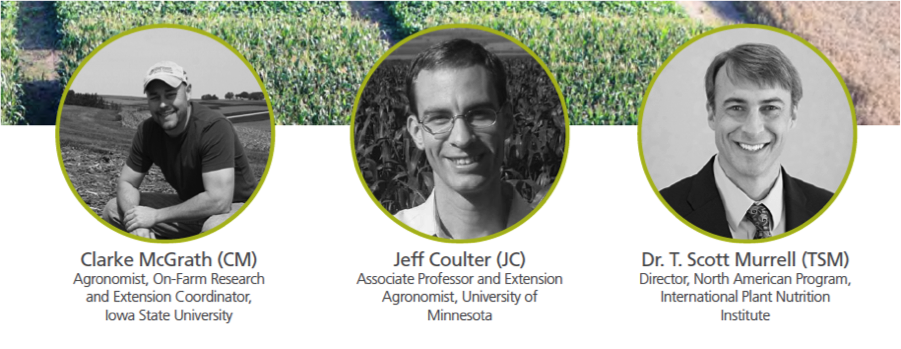
Q: What are the top quality-control considerations when setting up and running a test plot on your farm?
JC: One of the important things is to replicate your treatments. A minimum of three replications of your whole set of treatments is necessary. Four is better, and more than four is even better. That’s because sometimes something happens to one of the replications of the treatments, and you lose data from that plot. So you need another one. By the end of the study, you need to have a minimum of three or four good replications of data in order to perform good statistical data analysis.
(A replication includes all the treatments, including the control. A control or check treatment is one in which you haven’t used the product, so you have something to compare it to.)
If the purpose of the study is to simply determine the response of corn to nitrogen rate, for example, then you want to have a minimum of five rate treatments — if not six — within each replication. That number of rates allows you to develop a corn yield response curve. Basically, you plot the yield data in relation to N rate, fitting a line to it using regression analysis; then, based on the response curve you can calculate the economically optimum N rate. When doing a rate study, in addition to having a low-rate or zero-rate control plot, it’s also important to have a very high-rate plot as well. That gives you the full view of the response curve. You don’t want to have yield still increasing with the highest rate. At some point, you want to see where the yield plateaus.
CM: One thing we have to do is scout the plot regularly. There might be something strange that happens in one or two or more of the strips, whether it’s a population matter, insect issue, storm damage — you never know what could happen. And if you don’t pick up on it as it happens, come harvest time, you might not see it or recognize what happened, and it could skew results. If you go through the work of putting in the plots, you want to walk them on a regular basis; every week early in the season and every couple later on, so you know that the information is as genuine and as believable as possible.
Another thing is, if you’re going to do research, commit to doing it right. If you say, “I’ll do half the field this way, and the other half this other way,” in the end, you might have something, but to really do it right, you need to have replications. You do treated versus untreated, alternating over six or eight strips, if possible; four paired strips is usually considered a pretty solid test. You can’t just run a single side-by-side, or a few random strips. It has to be laid out and done with replications. If you’re going to do it, you need to commit to putting in the time and doing it right.
Q: What are the common pitfalls when doing on-farm research?
CM: It sounds really simple, but I sometimes find this a challenge: Keep things uniform. You do all the work to pick the right location; you get it planted, everything’s uniform, I’ve got my treatments overlaid; then, okay, I’ve got soybean aphids, or some late, emerging weed, so I have to spray my whole field. And then this happens — and it never fails; I’ve done it, so I know: I run through the field with something that’s got 80-, 100- or 120-foot booms and I put wheel tracks in some areas of my plot and not in others. It can happen so quickly; late-season spraying; you’re always going to run down a few beans or nip a few feet of corn here or there. On a field-wide basis, that’s one thing, but in a plot, that’s just 500 feet long, and I knock down 10 feet of a row in two places with my wheels, well, that can make a big difference in your results. We have to keep “uniformity” or “consistency” in mind so we don’t skew the data. For example, where I have to run a sprayer through the plots: Can I spray the plot perpendicular across all the reps so the tracks are equal across the entire area?
JC: If you don’t randomize the order of your treatments, that can get you into trouble. Say you’re doing your five treatments, and in the first set you do them in order, but in the next replication, you want to start with four, then number two, number five, then one, then number three. If you do all the treatments in all the replications in the same order, it can create a bias. Randomization also helps with biases that can occur due to equipment.
TSM: A successful experiment requires proper execution throughout the entire season. Prior to use, equipment needs to be properly maintained and calibrated, application maps and plot plans need to be done in advance, the logistics of putting out treatments and harvesting need to be thought out, and data need to be securely stored and readily retrievable.
A shortcoming of a lot of on-farm research is statistical analysis of the data collected. This requires special skills. Partnering with scientists and other trained personnel at universities or other organizations offers two important things to farmers and advisors interested in on-farm research: first, by ensuring the way treatments are arranged in the field adheres to statistical principles; and second, providing proper statistical analysis to determine if the yield differences observed are truly a signal above the background noise. Proper design makes proper analysis possible. Too often, people try to salvage data, unsuccessfully, from a poorly designed trial.
Q: What’s the best way to get a clear, actionable result from an on-farm test plot?
CM: I’ve noticed that people who are inquisitive can try to do things with too many variables. On the research farm, we have plot equipment and a lot of research technicians to help, so we can look at multiple variables. But when I go to work with farmers on their own farm, I suggest looking at just one variable. You need three or four replications of the same test. Equipment and acreage limitations make it so that you really can only look at one variable. Look at fungicide versus no fungicide, for instance, or seed treatment versus no seed treatment. If you throw another variable in, like a different plant population or different row spacing, all of sudden you need a lot more area, and that may mean that you’re including more soil types. It becomes pretty complex, so just look at one thing per test plot location.
JC: You have to start with a testable research question. You have to ask yourself, can I answer this question with the kind of trial I have in mind? If so, what do I need in order to do this? Make sure it’s accomplishable. It’s easy to get excited and to take on more than you really can. It’s always important to keep it manageable so that at the end of the year, you’ve got results.
Q: Should you always run your test strips in the same field, or do you want to look at multiple locations, or carry out your research over multiple growing seasons?
JC: When you’re doing a multi-location, or multi-location-year trial, then the question is, how do you look at the results? If you look at the overall average of all the trials, you’re looking at one set of numbers, but the average misses the extremes. In the real world, average is just average. It’s not really cutting it. We want to know what kind of responses we are getting in all conditions, so we can tailor our farming accordingly. If we are picking the average, we are hitting on average. But we are missing what is happening if it’s a wet year or a dry year. It’s important to dig into the data and spend time thinking about why the responses occurred. This can help one manage their fields on a more site-specific basis. Most growers are doing a pretty good job on average. But, if you start to manage on more of a site-specific basis, and put effort into the details, you’re fine-tuning it and can make improvements where needed. This can help you spend your dollars more wisely and get a greater return on your crop input investments.
CM: It depends. At the Armstrong Research Farm in Lewis, Iowa, we try to pick out uniform soil to get good data. Uniform soil tends to be on flat areas; long, gentle slopes; or high flats and plateaus. Test plots conducted on those types of ground produce data that applies well to those types of areas. But when you look at Iowa, especially southwest and south central Iowa, it has a lot of hillsides, terraces and contours. It’s not as uniform as you’d like. There’s a lot more variability of soils.
Growers will say, “You have plot results from uniform land that’s usually in pretty good shape, but I need to know what’s going to work on my rougher ground.” So, you’ll need to think about what questions you have, and what you’ll need to do to try and get the answers. If you are testing something like a seed treatment for disease or a foliar fungicide, it may be fairly uniform across a field, whether it’s good soil or not. So when growers look at the plot results, it will make sense to those with rough ground and those with uniform ground. But when testing things like plant populations or fertility, it may be that the results would be much different for the side of a hill, versus a high flat. So, you may have this great uniform chunk of ground to run a test plot, but it may not answer the questions you have for your rougher ground. There are always compromises when doing any on-farm research. When looking for answers on rougher spots, know it’ll be hard to get consistent soil in rougher ground. Just do your best to find a uniform area within those rougher areas, even if you have to do a fewer reps. You might not be able to put as much weight on the results, unless you do the plot over a couple of seasons, but you’ll start to get those answers.